At the latest Large Earth Convening, leaders from across the geospatial AI community joined forces to tackle the challenges of making foundation models accessible, practical, and impactful. Here’s how this collaborative group is shaping the future of Earth observation technology.
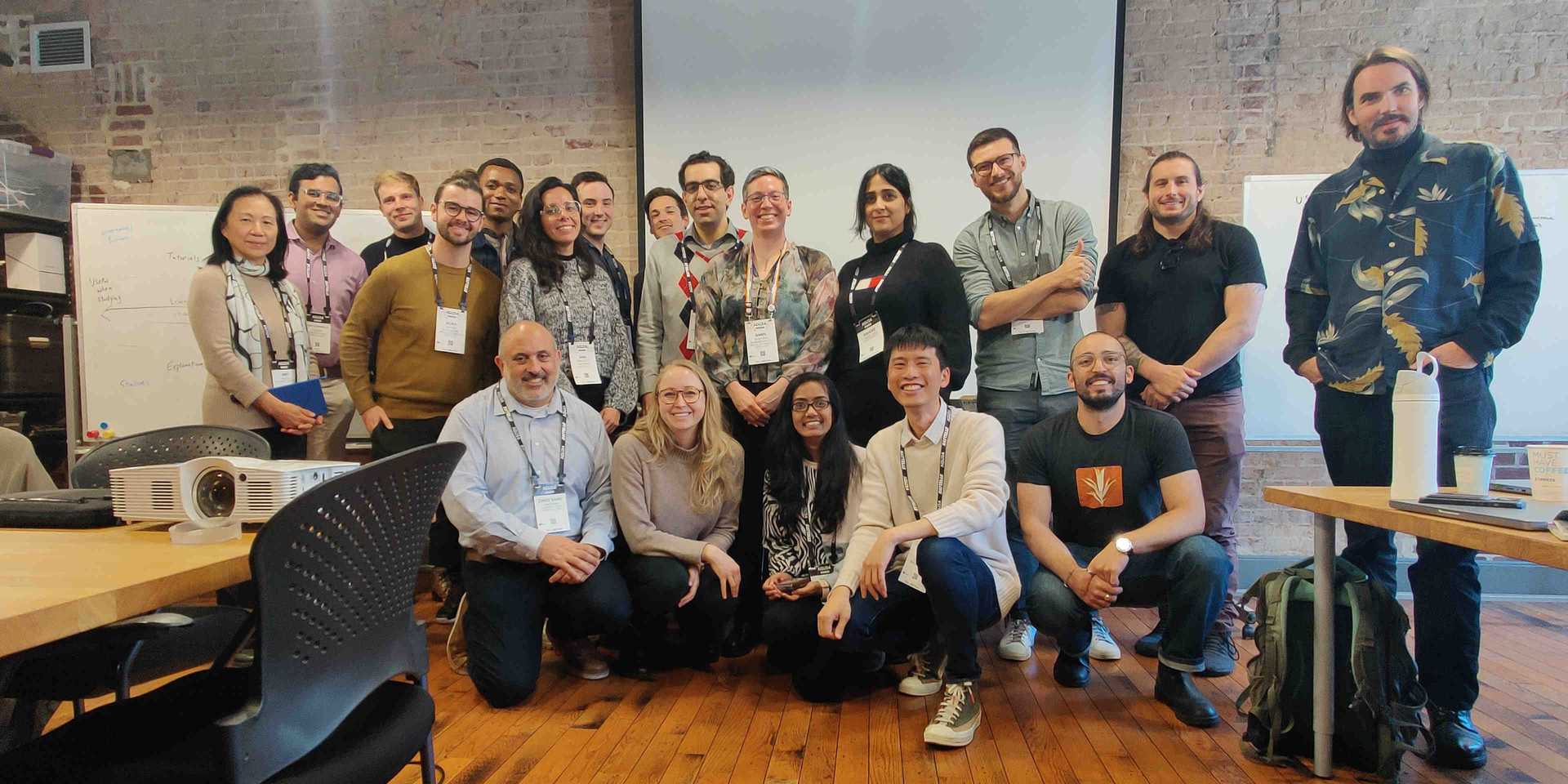
As we near the end of another transformative year in geospatial AI, our latest Large Earth Convening session brought together diverse minds to tackle a pressing question: How do we make Geospatial Foundation Models truly accessible to those who need them most? The confluence with AGU24 created a unique moment where academic insight and practical implementation sparked conversations that bridged the gap between theoretical advancement and real-world application.
The meeting included representatives from the following organizations (in alphabetical order):
- Clark University Center for Geospatial Analytics
- Development Seed
- Earth Genome
- NASA Harvest / Kerner Lab at Arizona State University
- NASA IMPACT
- IBM Research
- Spatial Informatics Group (SIG)
- WRI
Throughout our discussions, a realization emerged from practitioners: Foundation Models are a first-mile technology that requires a lot of last-mile effort to use practically. As some teams push the boundaries of model architectures – chasing multi-modal capabilities and extended time series analysis – others are asking fundamental questions about value and efficiency, wondering whether these resource-intensive models truly outperform simpler architectures like UNet in real-world applications.
This tension between innovation and practicality sparked three key initiatives for the coming year: creating an accessible survival guide for navigating the Foundation Model landscape, developing domain-specific benchmarks that speak to real-world needs, and establishing new ways to evaluate model quality through embedding representations. Each of these efforts reflects the community's commitment to bridging the gap between cutting-edge technology and practical implementation, ensuring that Foundation Models deliver genuine value to those working to understand and protect our planet.
Creating a Foundation Model Survival Guide
The landscape can feel dizzying for anyone stepping into the world of Geospatial Foundation Models. With new releases like NASA/IBM's Prithvi EO-2.0 and Clay v1.5 joining an already crowded field, practitioners face a maze of technical decisions: Which model architecture best suits their use case? How do they balance computational costs with performance needs? When is a simpler solution actually the better choice?
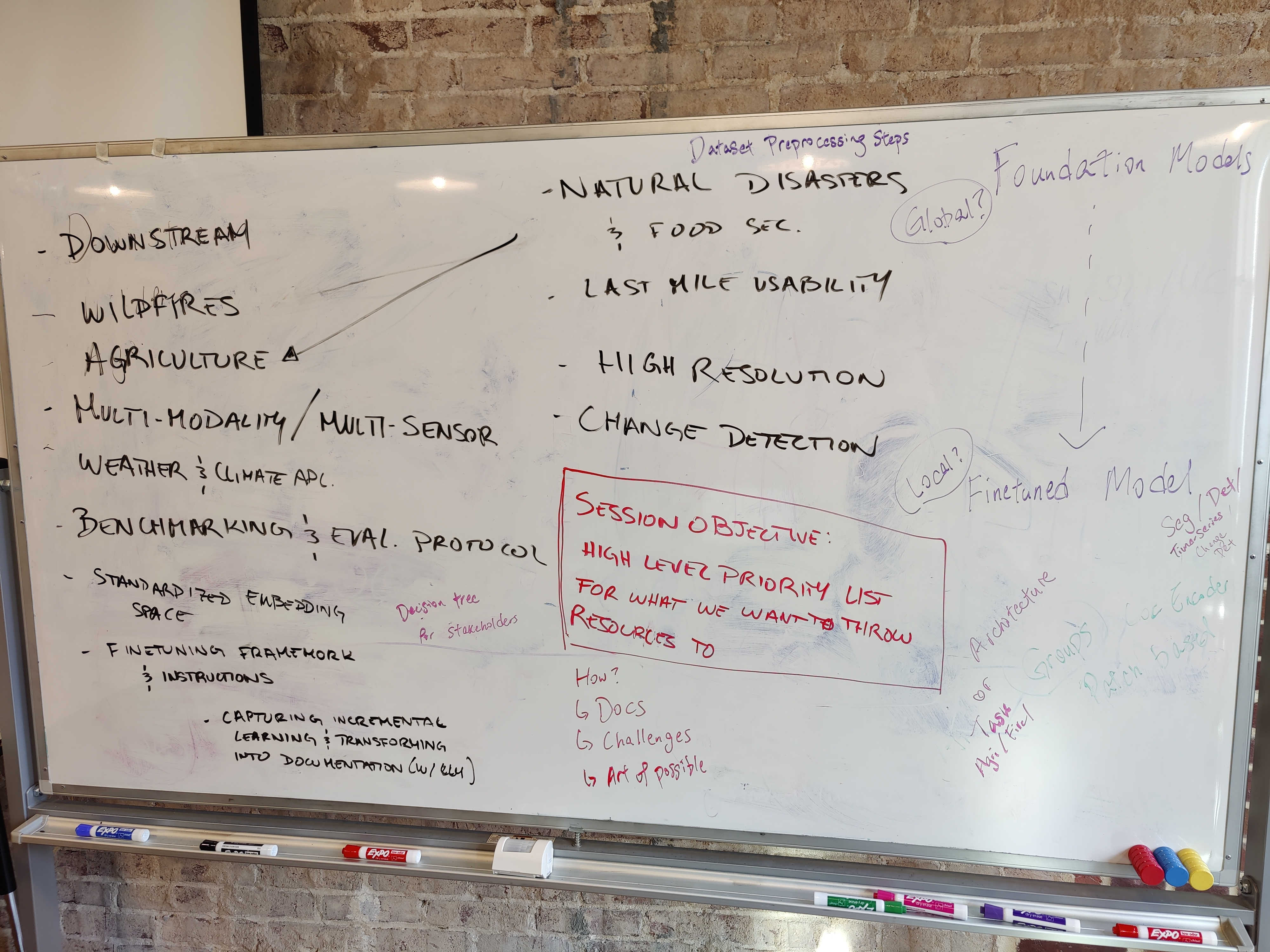
For example, you are a conservation organization needing to monitor deforestation across Southeast Asia. You are weighing whether to use Prithvi's powerful but computationally intensive architecture or opt for a lighter, pixel-based time-series model like Presto, and which downstream task is best suited for the desired output. The decision matrix involves both technical specifications and practical considerations like how to implement the downstream task, data availability, processing time, and deployment constraints – exactly the kind of real-world complexity a survival guide aims to address.
To bridge this knowledge gap, this sub-group will create a comprehensive guide that meets practitioners where they are. This guide will serve as a decision-making and implementation companion, structured around key personas and their unique needs:
For technical teams, we'll provide documentation of the typical downstream use cases for foundational models in remote sensing, such as model fine-tuning, zero or few-shot learning, and feature extraction, along with the technical steps to implement the use case. For project managers, we'll outline resource requirements and deployment considerations that impact project timelines and budgets. For funders and decision-makers, we'll offer clear frameworks for evaluating the real-world impact and operational costs of different approaches.
This guide will help teams build a technical implementation roadmap after they’ve used our GeoFM model selection guide to find the best model for their needs. It will be an open, living resource on GitHub, designed to evolve with community input and real-world experiences. We’re particularly excited about exploring ways to use language models to automatically capture the community’s incremental knowledge gains, as keeping this type of guide up to date for such a rapidly evolving field represents a distinct challenge.
From Universal to Domain-specific Benchmarks
For some time, there was this hope that creating proper, standardized benchmark datasets would allow for fair comparison of geospatial Foundation Models. Attempts such as GEO-Bench, PhilEO Bench, VLEO-Bench and more have tried to do this justice. Still, curating datasets that can cater to the diversity of model architectures is tricky since models may not be designed for certain sensor inputs (such as SAR data), time-series information, or location information.
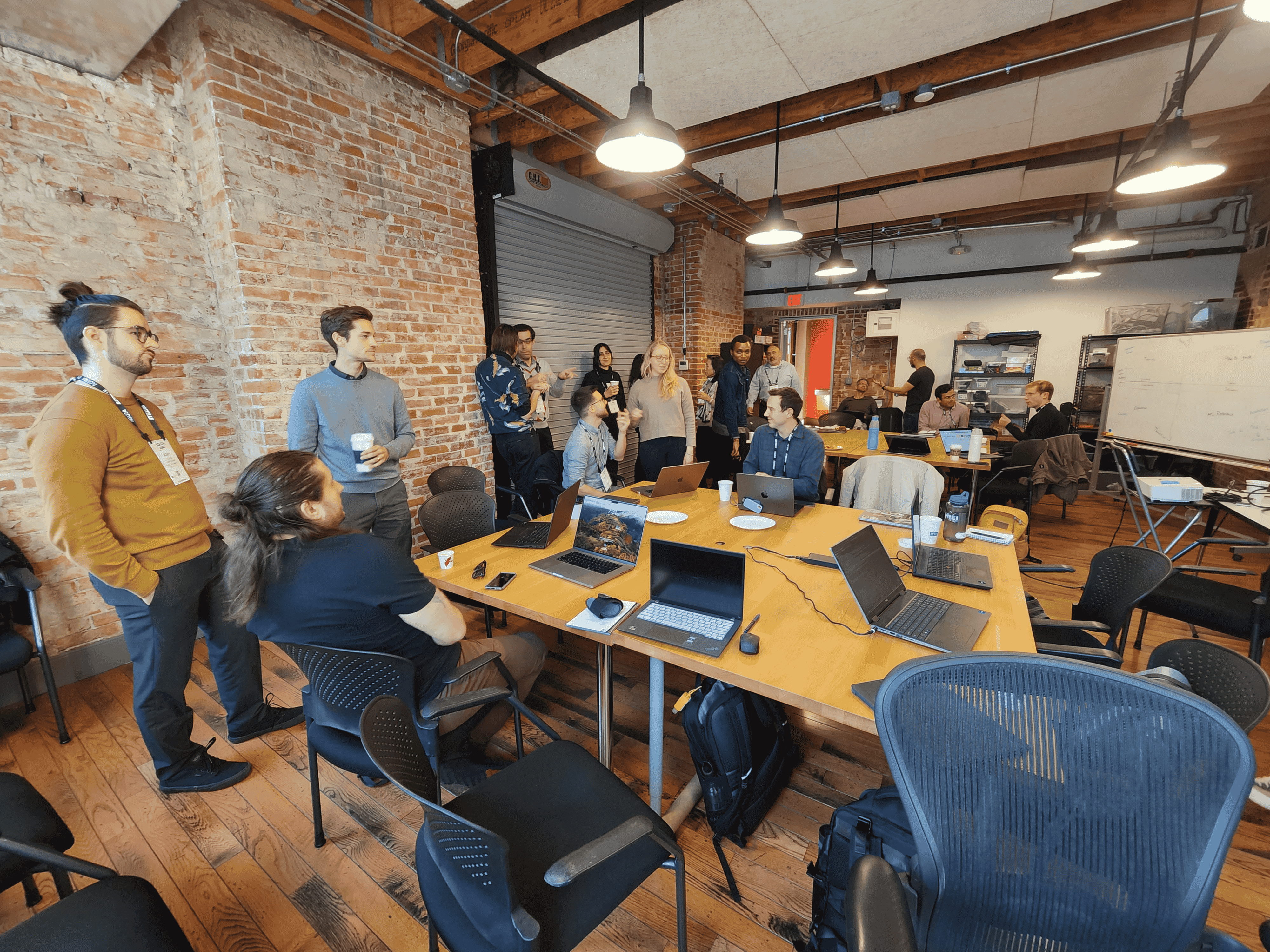
A fundamental challenge emerged in the divide between computer scientists (top-down) wanting easy-to-use benchmarks and domain scientists (bottom-up) interested in more nuanced use cases. A domain scientist interested in understanding hydrological flow may be cautious of adapting a Foundation Model benchmarked on burn severity, and having models that do well on land use land cover classification may mean nothing to those working on mapping geological minerals. Their definitions of "good enough" diverge significantly. This reality has pushed us to rethink our approach to benchmarking.
A new consensus emerged through our discussions: rather than forcing diverse use cases into a one-size-fits-all framework, we should embrace the rich variety of Earth observation applications. This shift mirrors the evolution we've seen in the broader machine learning community, where domain expertise increasingly shapes evaluation methods.
The PANGAEA benchmark offers an early glimpse of this approach in action. Instead of attempting to create a universal standard, it acknowledges the distinct needs of different applications while maintaining rigorous evaluation principles. This approach has already gained traction among domain scientists who previously viewed Foundation Models with skepticism. Everyone agrees that the tooling around applying benchmarks could be improved, and this will be a necessary condition for incentivizing domain scientists to provide the necessary expertise to drive the entire community forward.
Members of the group are planning to revive a proposal seeking funding to support this vision, focusing on creating the infrastructure needed to sustain community-driven benchmark development. We plan to launch a call for participation in early 2024, inviting domain experts across the Earth observation community to help shape these next-generation benchmarks.
Evaluating Foundation Models Based on the Quality of Embedding Representations
While domain-specific benchmarks get us closer to evaluating the performance of Foundation Models for common applications, they are still an incomplete proxy for the true power of Foundation Models. Ideally, there should be a way to capture the differences between Foundation Models based on a metric that is a priori of an idealized benchmark label dataset. The question is - how do we know if a Foundation Model has captured a representation of the world that is rich enough for multiple use cases?
One promising idea is to compare Foundation Models based on their embedding space geometry, based on the work in this ArXiV paper by Nomic AI. The benefit of such methods is that we only need to provide input data (e.g., satellite images) without their corresponding labels to perform inter-model comparisons. The main complication is that different Large Earth Foundation Models accept different inputs, and their output embedding lengths usually differ, too. It remains an open question on how the community can standardize data pre-processing steps and project their output embeddings into a comparable space to allow for such fair comparisons.
During the meeting, this sub-group interested in this FM embedding evaluation work decided to schedule monthly recurring meetings to discuss next steps, and we plan to share those details in the new year.
What's next?
The road ahead for Earth Foundation Models is both exciting and challenging. While we're making significant strides in technical capabilities, our community's strength lies in our ability to learn from each other and build together. Whether you're a researcher pushing the boundaries of new model architectures, a practitioner seeking practical applications, or a domain expert with unique insights, there's a place for you in this conversation. We'll be at the ESA-NASA International Workshop on AI Foundation Model for EO happening in May, let us know if you'll be there too. Join the working group or contact Ian Schuler, to stay in touch on what is happening, and help shape the future of geospatial AI.
Read more about our services and expertise in GeoAI here.
Related content
More for you
What we're doing.
Latest