Background
Mapping human and wildlife distributions
Conservation of wildlife is in a race against human expansion worldwide. With around 3% population growth annually in sub-Saharan Africa3, protecting wildlife and its habitats gets more and more difficult as humans move closer to wildlife areas through expansion of settlements, agricultural lands as well as raising livestock as main livelihood sources4.
Fundamentally, human-wildlife conflicts (HWC) are caused by competition for food and space. For the humans that live and work in close proximity to wildlife, wildlife activity can lead to loss of income, property, and sometimes human lives. This results in incidents where wildlife species of conservation interest are threatened or killed to prevent further conflicts5. An early warning system that can flag potential conflict on the ground will provide a significant guide to conservation practitioners, policy-makers, and local residents from designing policy intervention to loss prevention, and eventually minimise conflict. However, creating these HWP layers and warnings requires frequent monitoring and tracking of human, wildlife, and livestock activities and movements on the ground or from the air. Both camera trapping on the ground and aerial surveys from the air pose tremendous logistical challenges and costs. Both types of surveys produce image datasets which are prohibitively large to manually annotate, count, and record objects of interest. Instead, machine learning models can assist human annotators by generating high confidence predictions of images that contain objects of interest. This method may be more cost-effective for quickly locating human, wildlife, and livestock activities. In this study, funded by Microsoft AI for Earth and Global Wildlife Conservation, we proposed an AI-assisted aerial image analysis (AIAIA) approach to mapping human and wildlife distributions and potential conflicts in Tanzania.
We built an end-to-end AIAIA workflow that combines an AIAIA classifier and AIAIA object detectors to assist and guide human annotators to quickly and efficiently review images from aerial surveys. The binary AIAIA classifier acts as a filter that only keeps images that are likely to contain human activities, livestock, or wildlife. The three AIAIA object detectors for human activities, livestock, and wildlife detect, locate, classify, and count individual objects by subclass. These detections are used to create the Human-wildlife proximity (HWP) MVP visualization.
There is a subtle but important difference between HWC and human-wildlife proximity (HWP). HWC refers to direct conflicts between humans and wildlife, which require mitigation from conservation communities and policy makers. However, HWP reflects areas that could give rise to HWC in the long term, and HWP can be done through spatial analysis without loss assessment.
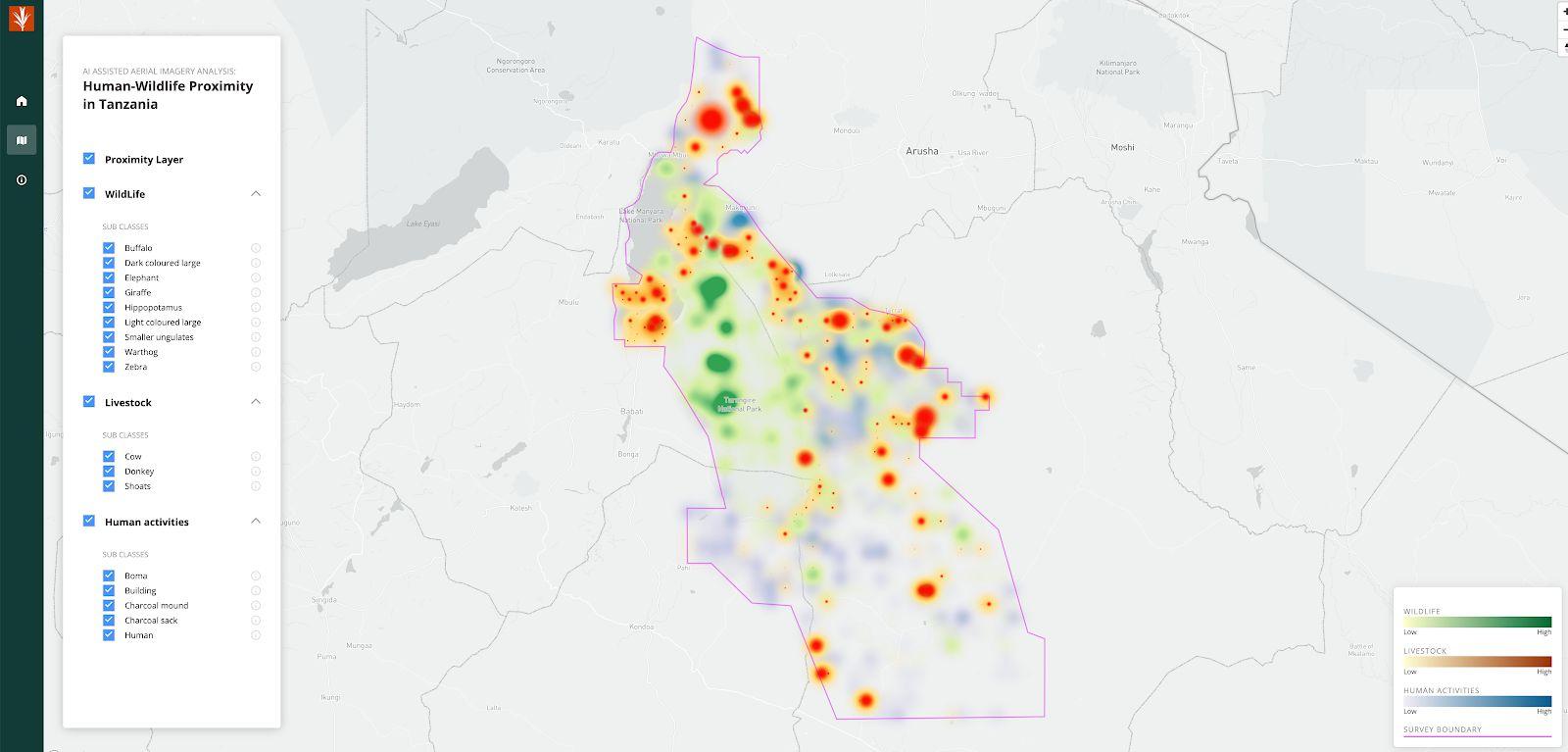